Synthetic Data Generation Services for AI and ML Applications
At Biz-Tech Analytics (BTA), we offer advanced synthetic data generation services, providing high-quality, custom datasets for AI and ML applications. Leveraging our expertise as a synthetic dataset generator, we create both structured and unstructured data, simulating real-world environments to optimize AI model training. Our solutions overcome the limitations of traditional data collection methods—addressing issues like errors, biases, privacy concerns, and security risks—while enhancing model performance. BTA’s synthetic data generation enables faster development, resolves data scarcity, and ensures compliance when handling sensitive or regulated data.
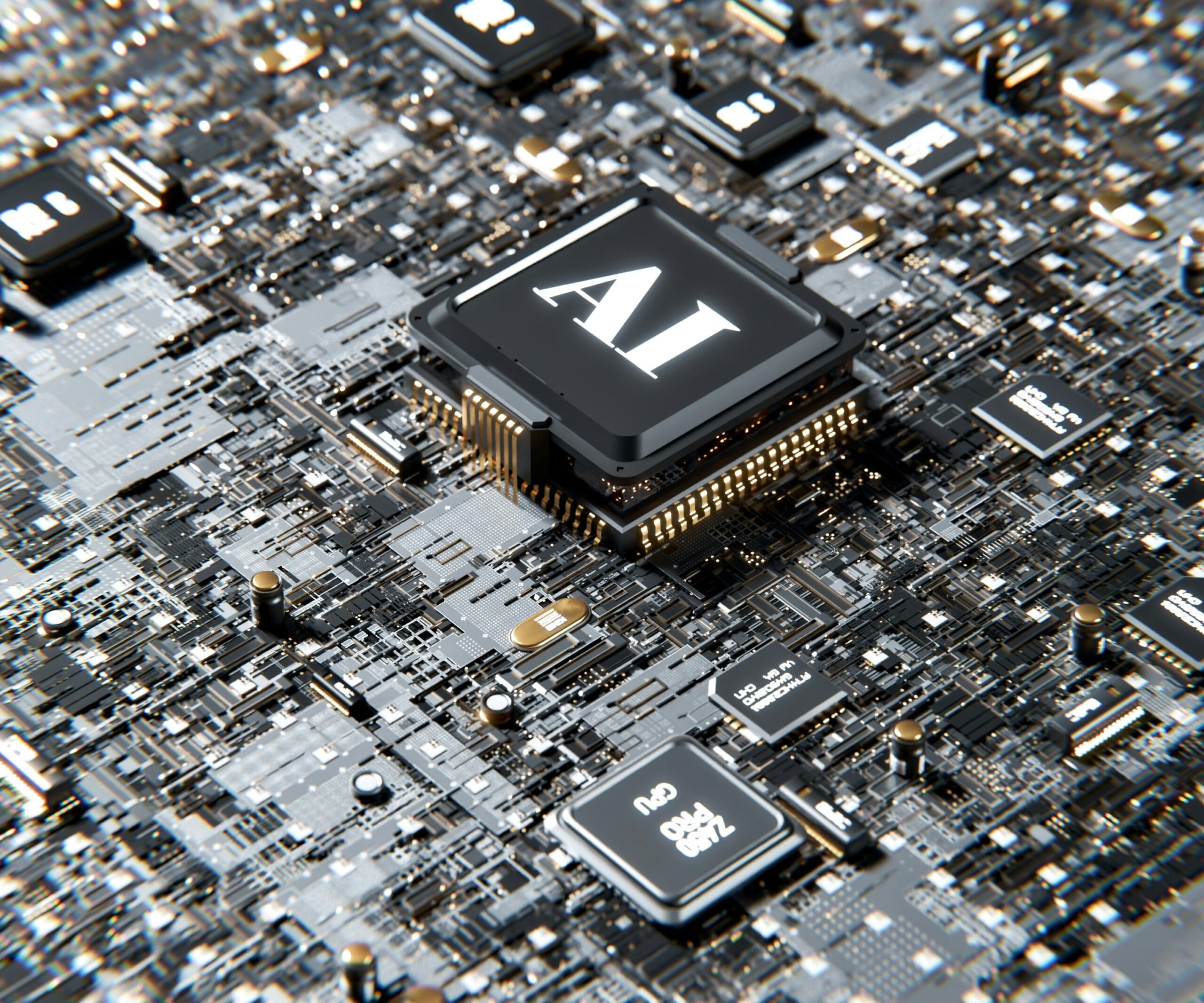
Our Clients
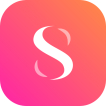
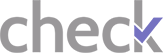
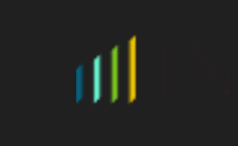
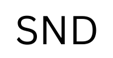

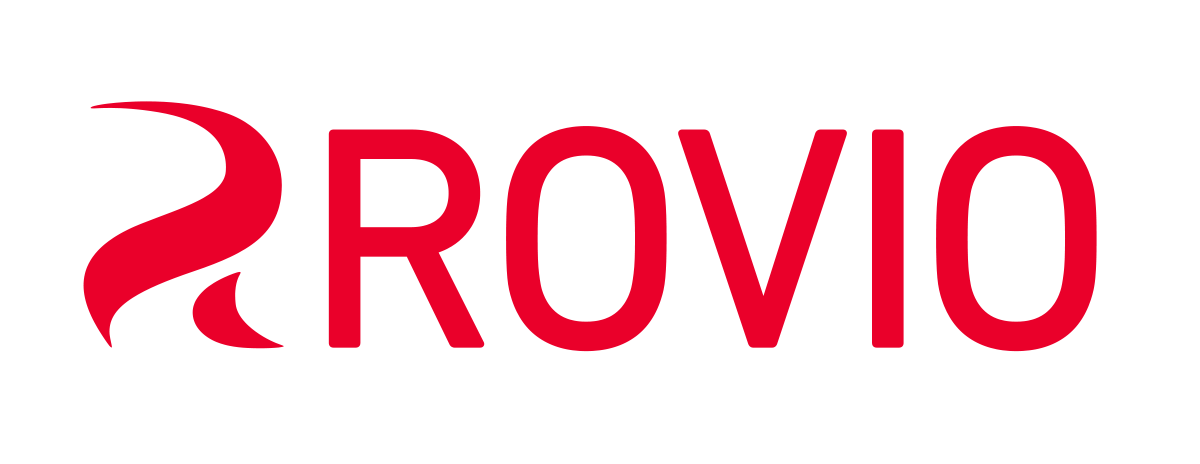
Success Metrics
What are Synthetic Data Generation Services?
At Biz-Tech Analytics (BTA), we specialize in synthetic data generation services, offering cutting-edge solutions that enable organizations to accelerate the development of AI and machine learning models. Synthetic data serves as a highly efficient alternative to real-world data, mitigating privacy concerns while closely mimicking actual data. By leveraging synthetic data for AI, businesses can enhance the performance of their models, ensuring accuracy and scalability across various applications.
Synthetic Data Services
Structured Data
(Tabular Data)
Unstructured Data
(Image, Video and Textual Data)
Beyond Real Data Limitations: Why is Synthetic Data Generation Important?
In the evolving landscape of artificial intelligence and machine learning, the ability to access and utilize high-quality data is crucial for success. However, real-world data often presents significant challenges, including scarcity, privacy concerns, and data biases. This is where synthetic data generation comes into play as a transformative solution, providing businesses with the tools they need to overcome these limitations.
At Biz-Tech Analytics (BTA), we recognize the importance of synthetic data for the future of AI development. Our synthetic dataset generator is designed to deliver high-quality, scalable, and secure data, enabling companies to push the boundaries of AI innovation. Here’s why synthetic data generation is so essential:
Accelerating AI and ML Development
Boosts Development Speed
Good-quality data for AI is essential for developing cutting-edge AI. By generating synthetic data tailored to the specific needs of a project, businesses can eliminate the delays associated with gathering and processing real-world datasets. This accelerates the software development lifecycle and brings AI models to market faster.
Reduces Costs
Traditional data collection, cleaning, and labeling are time-consuming and expensive. By leveraging data generation services, businesses can bypass these hurdles and minimize the costs of developing and maintaining high-performing AI models.
Supports Secure Research
Researchers often struggle with accessing and using large datasets due to strict data privacy regulations, which limit collaboration and innovation. Synthetic data, generated within secure research environments and enhanced by federated learning techniques, offers a solution by replicating the statistical properties of real data without exposing sensitive information. This approach enables researchers and developers to work with extensive datasets while maintaining compliance with data privacy laws, reducing the risk of privacy breaches, and allowing greater collaboration across teams and organizations. This ensures that data can be leveraged effectively for research without compromising privacy.
The Challenge of Real-World Data Acquisition
In the development of AI and machine learning models, acquiring real-world data presents several complexities and obstacles. Many industries, including healthcare, legal services, and finance, require comprehensive and accurate data. However, the lack of detailed datasets can significantly hinder the refinement of existing models, limiting their effectiveness and reliability. Here’s a breakdown of the primary challenges:
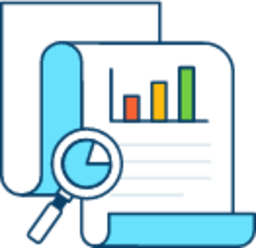
Data Scarcity
Sufficient and relevant data is often unavailable, especially in niche or emerging fields, leading to limited insights for model development.
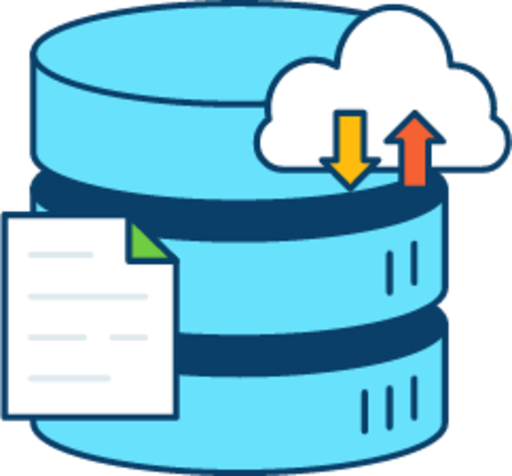
Unstructured Data
Much of the real-world data is unstructured, requiring significant time and effort to clean and organize before it can be utilized effectively.
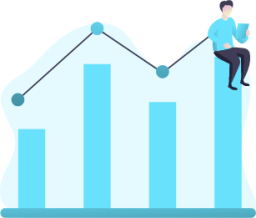
Data Bias
Real-world data is often biased, which can result in skewed AI models, reducing their accuracy and fairness in decision-making.
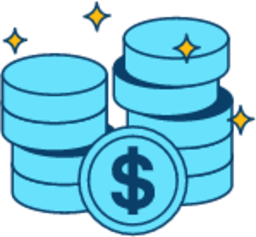
High Costs
Collecting, cleaning, and processing large datasets is resource-intensive, increasing the overall cost of AI and ML projects.
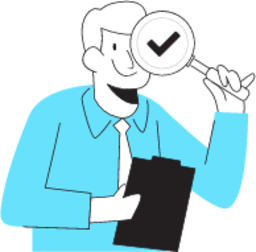
Data Quality Issues
Real-world datasets are frequently incomplete, inconsistent, or inaccurate, leading to poor model performance.
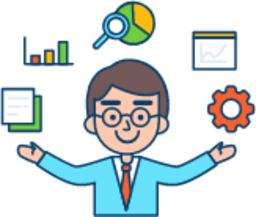
Integration Challenges
Bringing together diverse datasets from different sources for cohesive analysis is a time-consuming and labor-intensive process.
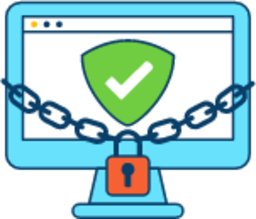
Privacy and Security Concerns
In sectors like healthcare and legal services, stringent regulations limit the collection of sensitive data, which can hinder the ability to gather and utilize information for research and analysis while ensuring compliance.
The Advantages of Synthetic Data
Synthetic data has emerged as a powerful alternative to real-world data, particularly for training AI and ML models. It offers a range of advantages that make it a crucial resource for innovation, model enhancement, and operational efficiency. Below are the key advantages of using synthetic data:
Cost-Effective Solution
Synthetic data eliminates the need for expensive data collection processes. It can be generated at scale, significantly reducing costs associated with obtaining and processing real-world data.
Enhanced Scalability and Agility
The ability to generate large datasets with various permutations and combinations allows for greater scalability. This flexibility enables AI-driven industries to adapt quickly to changing requirements without being constrained by data availability.
Data Availability and Use of Synthetic Data
Industries such as healthcare, finance, and legal services often face data privacy challenges that limit access to comprehensive datasets. Synthetic data provides a practical solution by generating large, high-quality datasets from a small subset of real data, ensuring compliance with regulations. This approach not only addresses privacy concerns but also facilitates the development of robust AI models for niche use cases where data is scarce, fostering innovation across sensitive fields.
Improved Data Annotation
The precise and accurate annotation of synthetic data ensures that datasets are well-balanced. This balance reduces bias, ensuring more equitable and fair model outcomes, ultimately enhancing task performance.
Enhanced Security and Privacy
With synthetic data, privacy concerns are mitigated as the data does not contain any personally identifiable information (PII). This eliminates the risk of breaching privacy regulations and ensures that sensitive data remains secure.
Simulation for Robust Models
Synthetic data enables the simulation of different scenarios, including rare or extreme cases. This helps in developing AI and ML models that are more robust and capable of handling a wider variety of situations.
Challenges and Limitations of Synthetic Data Generation
Data Quality and Accuracy
In healthcare, synthetic patient data may miss nuanced relationships between symptoms and diagnoses, potentially skewing predictive models.
Bias Amplification
If the training dataset has inherent biases, synthetic data can exacerbate these biases, affecting model fairness.
Complex Generation Process
The data generation process is intricate and requires advanced models, which can complicate operations and increase resource demands.
Lack of Metrics and Validation
Absence of standardized metrics and rigorous validation processes can undermine the reliability of synthetic data.
Resource Intensity and Ethical Concerns
Generating synthetic data is resource-intensive and may raise ethical issues related to data usage and privacy.
Standardization and Fidelity Issues
Challenges with standardization and maintaining data fidelity can affect the accuracy of synthetic data, particularly if statistical properties are not well-preserved.
Expertise Requirement
Without domain experts ensuring accurate statistical properties, biases may be introduced into synthetic datasets.
At Biz-Tech Analytics (BTA), our team of domain specialists adeptly navigates these challenges, ensuring the precision and reliability of our synthetic data solutions.
Synthetic Data for ML Model Training and Methods
The methods employed to generate synthetic training data are contingent upon the specific category of data that is required. From audio/visual data, numerical data, text, to categorical data.
Generative adversarial networks ( GANs)
The GANs method is used to generate image, video, and time series data for training AI models. Two neural networks, a discriminator and a generator, engage in an interplay where the generator fools the discriminator through adversarial training to produce realistic data. This technique is frequently employed to produce synthetic images for the purpose of training AI models.
Variational AutoEncoders (VAEs)
We frequently use these neural network architectures that compress and reconstruct data, allowing us to create both similar and new data points. Variational autoencoders not only create synthetic tabular data but are also used for image synthesis and data augmentation.
Data Augmentation
This involves transforming existing data, such as rotation and scaling, to create synthetic variations. It is primarily utilized in natural language processing (NLP) and for improving datasets in computer vision.
Statistical Modelling
This bootstrapping technique generates synthetic data from probability distributions based on observed data. Used for forecasting and financial modeling.
Procedural Content Generation (PCG)
The PCG technique uses algorithms to generate systematic, realistic, procedurally controlled data in virtual environments.
Reinforcement learning from Human Feedback (RLHF)
This approach uses human feedback to guide AI operations. Three stages comprise this methodology. During the pre-training phase, we train AI models on comprehensive datasets. In order to train the model, we gather human feedback, and human evaluators scrutinize the model to guarantee that it generates reliable outputs. Finally, during the reinforcement learning with feedback stage, we refine the AI model to align with human expectations.
Industry-Specific Applications: How Synthetic Data Services Transform Your Field
We leverage our AI training capabilities to revolutionize your business, equipping you for a better tomorrow with state-of-the-art applications. Our experienced professionals have partnered with clients across various industries to deliver top-tier AI data services tailored to their unique needs. Below are some of the groundbreaking projects we’ve successfully completed:
Healthcare
Researchers can generate data sets that facilitate more productive analytics by using precise and reliable data to train models. In addition, it facilitates innovation in clinical iterations.
Finance
Synthetic data generation produces anonymized datasets, facilitating the development of private and robust financial strategies. It uses data simulation to train models and improve their resilience.
Machine Learning Model Training
By using synthetic data, data scientists can enhance existing datasets, especially when real data is missing or limited.
Insurance
Synthetic data generates simulated claims for modeling diverse risk scenarios, aiding in the development of accurate and equitable policies while safeguarding privacy.
Automotive
Generative AI creates synthetic images of vehicles in various settings, allowing manufacturers to evaluate car performance without costly physical prototypes.
Retail
Retailers use synthetic images of clothing and merchandise to showcase products in different environments, eliminating the need for expensive photoshoots.
Gaming
Video game developers employ generative AI to create realistic environments and characters, enhancing gaming experiences without large teams of designers.
Product Design
Synthetic data helps businesses establish benchmarks and evaluate product performance in a controlled environment.
Behavioral Simulations
Synthetic data enables organizations to test hypotheses and validate models through simulations, without using original data.
Biz-Tech Analytics’s Approach: Here's how We Generate Synthetic Data
At Biz-Tech Analytics, we use a range of advanced techniques to generate synthetic data tailored to your specific needs. Our expert workforce ensures the creation of high-quality, industry-specific data, designed to meet the unique challenges of your sector. By incorporating Reinforcement Learning from Human Feedback (RLHF), we continually refine and optimize the data generation process, ensuring precision and efficiency at every stage.
We focus on delivering data solutions that not only meet but exceed your requirements. Whether it’s for training AI/ML models, developing new applications, or enhancing existing systems, our synthetic data is crafted to help you build robust, cutting-edge solutions. This approach allows us to offer highly accurate and cost-effective data, ensuring you can scale your AI/ML initiatives effectively while maintaining quality and performance.
Why Choose Us?
We collaborate with you to gain insight into all of your data-related needs and, as a result, demonstrate our effective workflow strategy. Subsequently, we establish a team to oversee the comprehensive execution plans of your organization. We provide a free sample of our customized workflow. That guarantees the project’s success with approval and mutual agreement on timelines! We ensure reliable and accurate data deliveries and create value for the brands that we work with. From STEM experts (Math, Physics, Biology, Chemistry, etc.) to marketing copywriters and linguists, from specialized programmers to manufacturing and scriptwriters, our USP at BTA is that we have employed domain-specific experts who are thorough masters in their respective fields.
FAQ Section
How accurate is synthetic data?
It is contingent upon the generation methodologies employed by a synthetic dataset generator. Replicating the statistical properties of actual data is imperative. Privacy concerns occasionally compromise the veracity of the synthetic data. Sophisticated synthetic data-generation techniques generate data that is both highly accurate and realistic.
What types of data can be generated through synthetic data generation services?
Data generation services can generate an endless list of data types, from tabular data to image data, from text to audiovisual data, and from behavioral data to genomic data to 3D data.
How does Biz-Tech Analytics's synthetic data generation service ensure the realism and quality of the generated data?
Our ability to collect data from a variety of regions and specialties guarantees that the data is relevant and of the highest quality. In addition to our expertise in the collection of historical data, we also conduct research to provide our clients with tailored solutions. We are cognizant of the potential hazards of handling sensitive data; consequently, we adhere to rigorous data security protocols.
How can synthetic data generated be used for training AI and machine learning models?
By addressing data scarcity, creating balanced datasets, and simulating various scenarios, synthetic data generation is highly useful for training AI and machine learning models. Validating models with synthetic data for machine learning encourages innovation. Synthetic data for AI trains AI models to be more robust and cutting-edge.
What is the difference between original data and synthetic data?
Original data is real-world data (RWD), which includes real-world characteristics and patterns and utilizes direct methods for data collection. Often, its collection is limited, highly sensitive, and necessitates safety protocols. Synthetic data is an artificial substitute that caters to all your data needs and applies that data to train AI and ML models, making them work efficiently and innovatively.